Deep neural networks are as good as practicing pathologists in classifying colorectal polyps, according to an experiment by a computer science and clinical research team led by Saeed Hassanpour, from the Dartmouth Cancer Center in Lebanon (New Hampshire). The team trained one such model on data from a single-center, and then checked its abilities in distinguishing the four most common colorectal polyps in imaging coming from 24 institutions in 13 states in the US, observing that its performance was comparable to that of practicing pathologists.
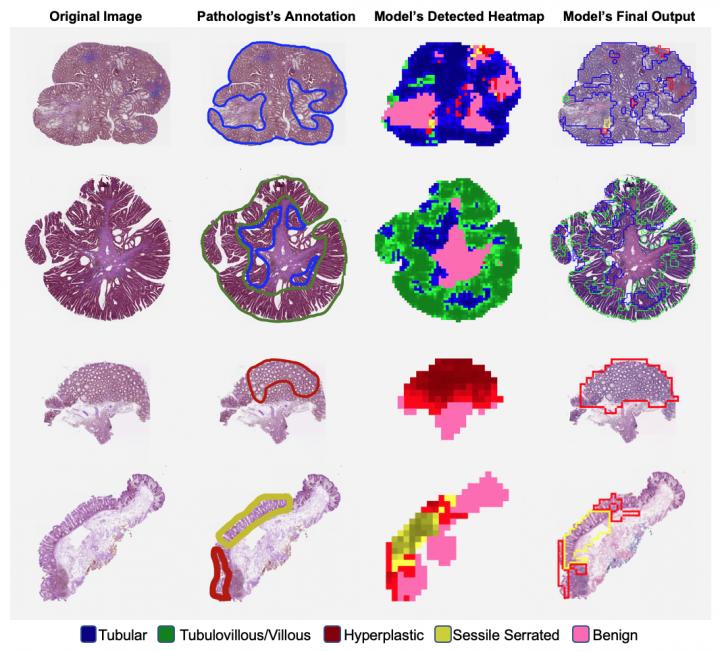
With permission of Saeed Hassanpour, PhD.
«To our knowledge, this study is the first to evaluate a deep neural network for colorectal polyp classification on a large multi-institutional data set with comparison with local diagnoses made at the point of care» Saeed Hassanpour and colleagues write in a paper just published in JAMA Network Open. They suggests that if the performance of the model will be confirmed in clinical trials, it could improve efficiency, reproducibility, and accuracy of the colonoscopy, the most common test used for colorectal cancer screening programs. «Early detection of cancer at an early, curable stage and removal of preinvasive adenomas or serrated lesions during this procedure are associated with a reduced mortality rate» the authors explain.
Hassanpour and colleagues used slides from Dartmouth-Hitchcock Medical Center from patients with tubular adenoma, tubulovillous or villous adenoma, hyperplastic polyp, and sessile serrated adenoma to train the deep neural network for their classification. On an internal data set, the mean accuracy of the model observed was 93.5%, compared with that of local pathologists equal to 91.4%. When tested on the external data set from 24 institutions (238 slides for 179 patients), the authors found that «the model performed at a similar level of accuracy, sensitivity, and specificity as local pathologists», (i.e. accuracy was 87.0% and 86.6% respectively). Furthermore, deep neural network and local pathologists had comparable confusion matrixes, which indicate similar misclassifications.
For the authors, the model, implemented in laboratory information systems, could guide pathologists, and «although expert practitioner confirmation of diagnoses will still be required, the model could help triage slides indicating diagnoses that are more likely to be preinvasive for subsequent review by pathologists». Authors want to evaluate their model in a clinical trial and to collect more data to improve its performance.