“Medicine cannot be learned quickly, because it is impossible for there to exist any established method in it, as for example when someone who has learned to write in one way that is taught then understands everything. Medicine from one moment to the next does things that are opposite, and it does opposite things for the same person, indeed, even things that are self-contradictory.”
The writer of the Hippocratic treatise On the Places in Man – written around the mid-fifth century BCE – articulated a dilemma of medical thought that has prevailed until today: How can a framework for medical theory and practice be built? Should medicine proceed based on the differences between patients or on their similarities?
The tension between the general and the specific continues in oncology today. In the past century, considering the general was the norm. Large trials generated evidence for treatments, under the brand of “evidence-based medicine”, the results of which were then applied across the patient population. But not everyone is the same. In the past twenty years, personalised medicine, with its premise of tailoring treatment to a patient’s individual profile of mutations, entered oncology. The hype and hope with which personalised medicine – variously also called precision medicine or stratified medicine – has been greeted suggested it may revolutionise cancer treatment. But will it?
Hype or hypothesis?
One of precision oncology’s most prominent critics, Vinay Prasad, haematologist-oncologist and Associate Professor of Medicine at Oregon Health and Science University, has called precision oncology “a hypothesis in need of verification,” (Nature 2016, 537:S63). Damian Rieke, oncologist at the Charité University Hospital in Berlin, Germany, expresses his reservations more cautiously: “I do believe that precision oncology has a future and that it makes sense to continue to pursue this approach. But we have to be careful not to throw evidence-based medicine overboard, just because we can now sequence our patients.”
The starting signal for precision oncology was the development of imatinib as a treatment for chronic myeloid leukaemia, CML. Imatinib inhibits the bcr-abl kinase, the aberrant protein driving CML. In the phase I trial of imatinib, 53 of 54 patients went into complete haematological response. This created a new paradigm of targeted treatments that take aim at driver mutations. But with the benefit of hindsight, commentators point out, imatinib and its effectiveness in CML turned out to be an exception. In fact, imatinib is one of the very few targeted agents that achieve a long-lasting benefit, even when administered on its own. For many other precision oncology agents, this success was not repeatable. Tumour heterogeneity and clonal evolution usually give cancer multiple escape routes from targeted therapy.
Hence, treatment response and prognosis is much less predictable by genetic tests than was expected based on the imatinib-CML paradigm. And while the course of illnesses with a clear, identifiable, singular genetic alteration, such as CML, may be altered dramatically, it is much less clear whether this promise holds true for other cancers (Lancet Oncol 2016, 17:e81–86). In 2016, Prasad noted that, “When patients with diverse, relapsed cancers are given drugs based on biological markers, only around 30% respond at all and the median survival rate is just 5.7 months.” He estimated that “precision oncology will benefit around 1.5% of patients with relapsed and refractory solid tumours” (Nature 2016, 537:S63).
Making sense of complexity
The main difference between evidence-based medicine and precision medicine is the depth of data, says Rieke. “When a patient comes to the clinic, we try to gather as much data as possible – diagnosis, stage, previous treatments, lifestyle, allergies – and then decide what the best therapy is, based on the currently available data… When [in addition] we sequence 10,000 genes, we have many more datapoints and a higher complexity. In both approaches, we personalise treatment based on the patient, but in precision oncology, we have the added genetic datapoints.”
Molecular tumour boards attempt to make sense of this complexity. “At the Charité, two to three doctors work full time to look at patients’ genetic data and comb through the literature. This information is collected and assessed, based on study design and the level of evidence. The aim is to make the recommendation for which there is the best evidence”, explains Rieke.
Rieke led a study comparing the treatment recommendations made by molecular tumour boards (MTBs) worldwide (JCO Precision Oncology 2018, 2:1–14). The team sent genomic information of four fictional patients to MTBs. Although all MTBs received the same information, the recommendations of the five MTBs that replied differed substantially for some patients. This is a problem, says Rieke. “On the one hand, it means we create a lot of evidence which is not optimally retrievable for the individual patient. On the other hand, we have no standards for how to handle genetic data.”
All MTBs received the same information, but the recommendations of those that replied differed substantially for some patients
One step towards harmonising recommendations is to make genetic evidence more readily accessible. A dozen knowledge bases store evidence on genetic mutations, of which Rieke estimates only a few to be useful, “but each database also holds unique information – this shows us just how much data we have.”
A framework for aggregating and harmonising clinical interpretations of detected variants has now been developed using data from six prominent cancer variant knowledgebases (biorxiv.org/content/10.1101/366856v1.full.pdf). The framework aims to provide access to concise, standardised, and searchable clinical interpretations (therapeutic, diagnostic, prognostic and predisposition) of detected variants drawn from across multiple institutions that gather and store that data. The harmonised interpretations from those six knowledgebases have been published on an open and searchable website search.cancervariants.org.
Therapeutic freedom versus treatment algorithms
How to handle genetic data and make treatment decisions based on – eventually harmonised – genetic evidence is a different debate. Rieke advocates giving oncologists freedom to decide.
“I think we are far removed from assigning optimal treatments using a computer. We shouldn’t treat patients according to an algorithm, but based on experience – taking into account, for example, previous therapies. Oncologists should have a certain therapeutic freedom, but the available evidence should be the same – which it isn’t.”
Developing treatment algorithms is hard, not least because statistical considerations have to be taken into account. Jan Bogaerts, Scientific Director at Europe’s largest independent cancer research organisation EORTC, explains the dilemma: “I’m concerned about which methodology we will use to tease apart the changes we make for patients, and worry about the multiplicity of testing. The more research claims to be personalised, the smaller the subclasses of analysis will become. One idea is to solve this with AI, but these methods are very data hungry – I’m not sure that will work with the data we have available.”
“An approach in which we do withinpatient experimentation before deciding on a treatment would improve the situation a lot”
Several years ago, Bogaerts was asked to comment on an idea of comparing several treatment assignment methods in parallel – but this approach had to be abandoned as the statistical requirements were too complex. “If we try to compare two or more gene-based methods of deciding which patient gets which treatment, and want to answer the question of which one of ten drugs should be given to a patient, we have a very difficult problem. In our answer, we would mix the relative efficiency of drugs tested and the way of assigning patients to them. In reality, we would only be able to give a pragmatic answer, that one method of assigning patients gives a somewhat better survival, without being able to identify the reasons why that happened.”
From a statistician’s point of view, Bogaerts sees a protocol in which each patient serves as her or his own control as ideal. “For statisticians, an approach in which we do within-patient experimentation before deciding on a treatment would improve the situation a lot. But this is totally utopian in most situations in cancer.”
The VICC Meta-Knowledgebase
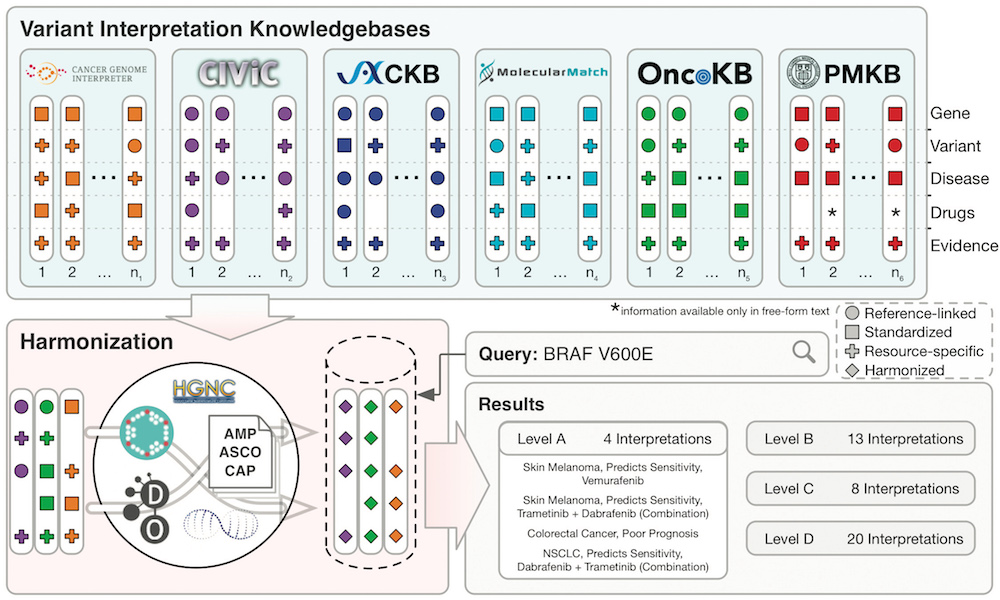
The Variant Interpretation for Cancer Consortium (VICC) meta-knowledgebase is a harmonised collection of clinical variant interpretations and related variant information informing of the clinical significance of variants observed in human cancers. The meta-knowledgebase was created to evaluate the disparities in variant interpretation content and structure across established resources of clinical interpretation knowledge. It harmonised information from six prominent cancer variant knowledgebases: Cancer Genome Interpreter, CIViC, CKB, Molecule Match , OncoKB () and PMKB (pmkb.weill.cornell.edu).
The VICC meta-knowledge base is searchable using its associated web interface: search.cancervariants.org
Precision oncology on trial
Clinical trials put precision oncology to the test. The French SHIVA01 trial was the first, and so far only, randomised trial of therapy directed at pathway mutations. In this study, patients with metastatic or refractory solid tumours who had already received the approved line of treatment, including molecularly targeted agents, were randomly assigned to either receive treatment aimed at the pathway in which their molecular alteration fitted, or to receive treatment as per clinician’s choice. The primary endpoint in the study was progression-free survival. The results showed that treating patients according to the molecular pathway did not improve median progression-free survival.
For Rieke, the design of the study is partly to blame. “SHIVA01 was too simplistic, it targeted only the signalling pathways in which a genetic alteration is placed. However, there are more specific inhibitors that target the exact mutations causing a pathway activation. While thinking in terms of pathways is already more personalised than conventional therapy, the assignment of therapy was obviously not good enough.”
Prasad, too, readily grants that these results do not mean that precision oncology per se will fail, just that the tested strategy failed. But he warns that “… because the tested strategy is consistent with the growing off-protocol use of these drugs, results of the SHIVA trial should serve as a powerful deterrent against the off-protocol use of unapproved targeted drugs…” (Lancet Oncology 2016 17:e81-e86).
Concerns about the extent to which these drugs are being used off protocol were highlighted in a recent article that looked at ‘Early Returns from the Era of Precision Medicine’, principally from a cost-effectiveness standpoint (JAMA 2020, 323:109–110). “Off-label use has been estimated as high as 30% of use for some anticancer agents,” notes the author. A key issue, he says, is that anticancer drugs tend to be tested in metastatic cases first, “because clinical trial recruitment is easier and the time from initiation of therapy to a meaningful end point is shorter.” However they are then sometimes used off-label to treat earlier-stage cancers or certain other cancer types, he notes, “even before clinical trials are conducted or completed”.
Maud Kamal, scientific manager in charge of precision medicine project coordination at the Institut Curie, in Paris, and scientific coordinator of the SHIVA01 trial, sees validation in the trial’s secondary endpoint. “As a secondary endpoint, we used patients as their own control. We compared progression-free survival of patients undergoing targeted therapy as compared to conventional therapy and see that a subpopulation of patients does better if they are treated based on the altered pathway. So treating based on alteration works in a subpopulation of patients.”
Kamal acknowledges room for improvement. “We reassessed the SHIVA01 results by classifying the alterations used in the SHIVA01 algorithm according to the ESCAT scale, which classifies the association between a molecular alteration and a specific targeted therapy. We found that the majority of alterations in the algorithm were tier 3 alterations, for which there is only moderate evidence. We may need to refine the algorithm to give better treatments to our patients.”
Kamal will keep looking for a proof for the precision oncology approach. “We will need more trials with different types of design to have clinical proof. As for the treatment algorithms, we need to be precise. While one mutation may be actionable, another mutation in the same gene but not at the same position might not be actionable and targetable to the same degree. We know clinical evidence is important, so we should not just stick our treatment decision on a gene or signalling pathway, but go beyond and look at the specific alteration.”
Real world data cannot replace RCTs
What can be done to increasingly tailor cancer treatment to the patient, but maintain the “safety in numbers” given by large trials? The collection of so-called real world data after drug approval may not cut it, says Bogaerts. “I’m worried that, guided by hype, and without sufficient certainty that the precision oncology method will work, we will give up parts of randomised clinical research and bank a lot on real world data.
“But this data is not necessarily geared towards answering our questions. If precision oncology doesn’t come through as promised, we will have big gaps in our knowledge in the future.”
In January 2019, the EORTC launched its ‘Manifesto for establishing treatment optimisation as part of personalized medicine development’, (bit.ly/EORTC_Treatment-Optimisation-Manifesto, see also p9). This envisages an approach in which relevant questions for patients outside the, often-limited, patient group included in a trial are answered before approval, Bogaerts explains. “In the current model, we test a restricted number of almost ideal cases and then approach all the other cases – often the majority – by hand waving, saying ‘we will see in the clinic how we will solve this small problem.’ Then a cancer patient comes to the clinic who doesn’t exactly fit the description of patients in the trial for which the drug was approved. What do we do with this patient?
“The manifesto asks drug developers to research this question up front. We should move to a situation where we have broader research into how a drug is going to be used once it is approved, and talk about the practical problems clinicians will have when applying this drug later on.”
Rieke echoes this caution. “Just because we now have genetic datapoints, we shouldn’t throw overboard everything we’ve learned in the past fifty years. We shouldn’t think that this method is better per se – because it isn’t, not yet.”
And he offers an answer to the age-old question of: What would you do, doc? “If I had a tumour, I would probably have it sequenced. But if we don’t find anything for which there is good evidence, I would decide on having a conventional therapy that is well understood. In this case, evidence beats biological hope.”